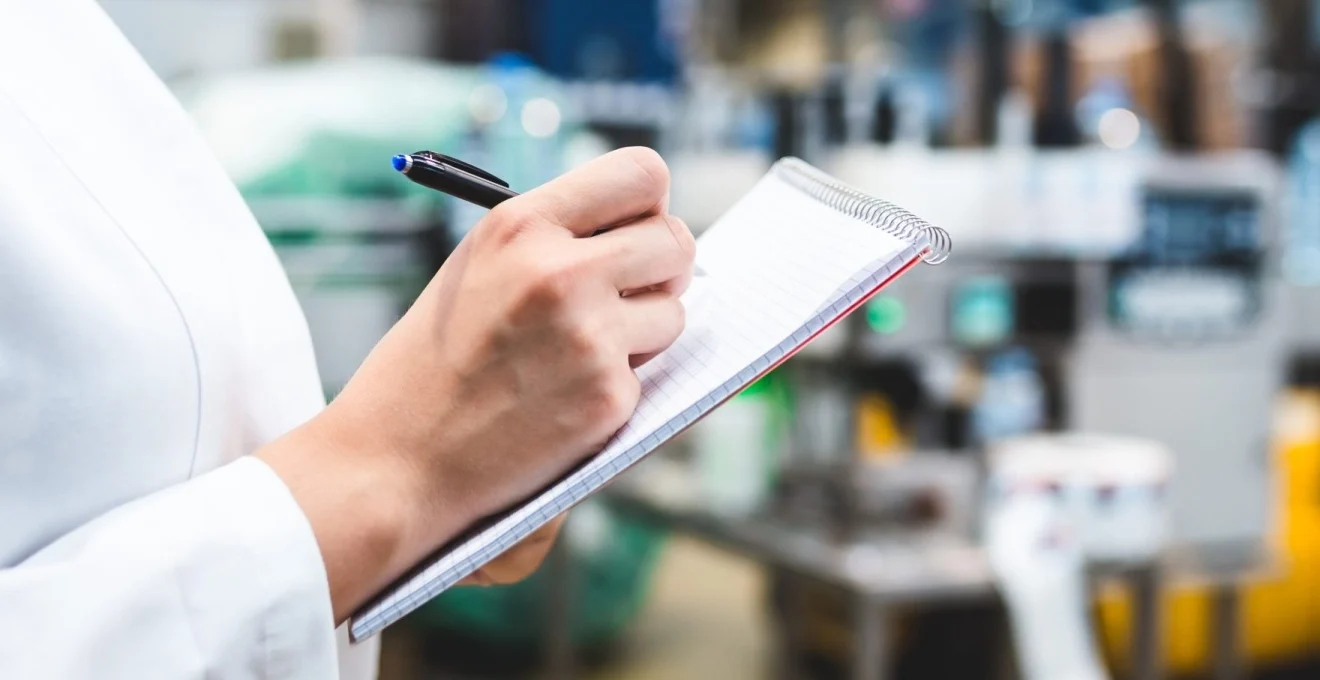
Quality control has entered a new era where advanced imaging technologies are completely transforming how manufacturers detect defects, ensure consistency, and maintain product excellence. The integration of artificial intelligence, sophisticated camera systems, and automated inspection processes is enabling unprecedented levels of precision and efficiency. These technological innovations are not merely incremental improvements—they represent a fundamental shift in how quality assurance operates across industries from automotive and electronics to pharmaceuticals and food production.
The financial implications are significant, with modern imaging solutions reducing inspection costs by up to 80% while simultaneously improving detection rates for even microscopic defects. For manufacturing facilities processing thousands of units daily, the ability to catch quality issues in real-time before they result in costly recalls or reputation damage has become a competitive necessity rather than a luxury.
As production speeds increase and consumer expectations for perfect products rise, traditional human-based inspection methods simply cannot keep pace. This technological revolution in quality control is creating opportunities for manufacturers to not only improve their bottom line but also to gain valuable insights from the massive amounts of visual data being collected throughout their production processes.
Machine vision systems for Real-Time quality inspection
Machine vision systems represent the cornerstone of modern quality control, providing manufacturers with the ability to inspect products at high speeds with incredible accuracy. These systems combine specialized cameras, lighting, and sophisticated software algorithms to detect and classify defects that might be impossible for human inspectors to identify consistently. The integration of machine vision into production lines enables 100% inspection rather than the statistical sampling approaches of the past.
The core functionality of these systems involves capturing high-resolution images of products as they move through production, analyzing those images against predetermined quality parameters, and making instant pass/fail decisions. Advanced systems can evaluate multiple quality attributes simultaneously, from dimensional accuracy and surface defects to color consistency and component placement.
What makes modern machine vision particularly powerful is its ability to adapt to different products and conditions. Using machine learning techniques, these systems can be trained to recognize new defect types without extensive reprogramming. This flexibility allows manufacturers to quickly adjust their quality control processes as products evolve or as new quality issues are identified.
The adoption of machine vision has accelerated dramatically, with the global machine vision market expected to reach $18.5 billion by 2028, growing at a CAGR of 10.2%. This growth reflects the increasing recognition that automated visual inspection is not just about detecting defects—it's about gathering actionable data that can help optimize the entire manufacturing process.
Hyperspectral imaging analysis with NIR and SWIR cameras
Hyperspectral imaging represents one of the most sophisticated approaches to quality control, capturing information across the electromagnetic spectrum that is invisible to both human eyes and conventional cameras. By utilizing Near-Infrared (NIR) and Short-Wave Infrared (SWIR) wavelengths, these systems can detect chemical composition, moisture content, and internal structural issues that would otherwise remain hidden.
The technology works by breaking down the light reflected from an object into hundreds of narrow spectral bands, creating what is essentially a chemical fingerprint of the material. Each pixel in a hyperspectral image contains a complete spectral signature, allowing for unprecedented material identification and analysis. This capability is particularly valuable for industries where internal quality matters as much as external appearance.
In food processing, for example, hyperspectral systems can detect contaminants, assess ripeness, or identify spoilage that appears perfectly normal to visual inspection. Pharmaceutical manufacturers use these technologies to verify the chemical composition of medications, ensuring that active ingredients are properly distributed throughout tablets or capsules.
The integration of hyperspectral imaging into quality control workflows has reduced product recalls by up to 35% in pharmaceutical manufacturing, where chemical composition verification is critical to patient safety.
The implementation of hyperspectral imaging does require specialized expertise, as the massive amounts of spectral data generated need sophisticated algorithms to interpret meaningfully. Many manufacturers partner with specialists like claravision.com to develop customized hyperspectral solutions that address their specific quality control challenges.
Deep learning defect detection with YOLO v5 and RetinaNet
The integration of deep learning algorithms into machine vision systems has dramatically improved defect detection capabilities. Among the most powerful technologies in this space are YOLO v5 (You Only Look Once, version 5) and RetinaNet, neural network architectures specifically designed for fast and accurate object detection. These frameworks have revolutionized how manufacturers approach automated quality control.
YOLO v5 processes entire images in a single pass through the neural network, making it exceptionally fast for real-time applications. It can detect and classify multiple defect types simultaneously, often operating at speeds of 30-60 frames per second even on standard hardware. This performance makes it ideal for high-speed production environments where products might be moving past inspection points in milliseconds.
RetinaNet, meanwhile, excels at detecting small defects that might be missed by other algorithms. Its focal loss function gives greater weight to hard-to-detect defects during the training process, resulting in higher sensitivity for subtle quality issues like hairline cracks, minor discolorations, or tiny missing components.
Training these deep learning models requires substantial datasets of defect images, which can present challenges for manufacturers just beginning their quality control automation journey. However, techniques like transfer learning and data augmentation have made it possible to develop effective models with fewer examples. Many systems can now be trained with just hundreds of defect images rather than the thousands previously required.
Deep Learning Model | Detection Speed | Accuracy | Best For |
---|---|---|---|
YOLO v5 | 30-60 FPS | 85-95% | High-speed production, multiple defect types |
RetinaNet | 15-30 FPS | 90-98% | Small defects, high precision requirements |
Automated pattern recognition using OpenCV and TensorFlow
For manufacturers implementing computer vision quality control systems without extensive AI expertise, open-source libraries like OpenCV and TensorFlow provide accessible entry points. These powerful software frameworks offer pre-built functions for image processing, pattern recognition, and machine learning that can be adapted to specific quality control applications.
OpenCV (Open Source Computer Vision Library) provides tools for basic image manipulation, feature detection, and traditional computer vision algorithms. It's particularly useful for applications where defects have clear visual characteristics—like checking for proper alignment, verifying dimensions, or detecting obvious surface flaws. The library's wide adoption means there's extensive documentation and community support available for quality control engineers.
TensorFlow, developed by Google, offers more sophisticated deep learning capabilities while still being accessible to developers without specialized AI backgrounds. Its high-level APIs like Keras allow for relatively straightforward implementation of neural networks that can learn to recognize complex defect patterns from training data.
Many manufacturers implement a hybrid approach, using OpenCV for preprocessing images (enhancing contrast, removing noise, isolating regions of interest) before passing them to TensorFlow models for more sophisticated analysis. This combination leverages the strengths of both traditional computer vision techniques and modern deep learning approaches.
The cv2.matchTemplate()
function in OpenCV, for example, can quickly verify that product labels are correctly positioned, while a TensorFlow model might simultaneously check that the text on those labels is accurate and readable. This layered approach to quality inspection provides comprehensive coverage while optimizing computational resources.
Multi-angle inspection with photometric stereo techniques
Surface defects like scratches, dents, or texture inconsistencies often appear differently—or become entirely invisible—depending on lighting conditions and viewing angles. Multi-angle inspection systems address this challenge by capturing and analyzing products from multiple perspectives simultaneously, ensuring that defects can't hide from detection.
Photometric stereo techniques take this concept further by using controlled lighting from different angles while the camera position remains fixed. By analyzing how surfaces reflect light from various directions, these systems can construct detailed 3D surface maps that reveal even the subtlest texture anomalies. This approach is particularly effective for inspecting highly reflective surfaces like automotive paint, metal components, or glass products.
Modern photometric stereo systems can detect surface variations as small as 50 microns—about the width of a human hair—making them invaluable for industries with stringent finish quality requirements. Automotive manufacturers use these systems to verify paint quality and detect almost imperceptible dents that might only become visible to customers under specific lighting conditions.
The computational requirements for multi-angle inspection are higher than for single-view systems, but the dramatic improvement in defect detection rates—often increasing catch rates by 30-40% for certain defect types—more than justifies the investment for many manufacturers. As processing power continues to become more affordable, these sophisticated inspection approaches are becoming accessible to smaller manufacturers as well.
Industrial camera technologies transforming QC workflows
The evolution of industrial camera technologies has been a primary driver in the advancement of automated quality control. Today's industrial cameras offer resolution, speed, and sensitivity levels that were unimaginable just a decade ago, enabling inspection processes that can keep pace with even the fastest production lines while detecting increasingly subtle defects.
Modern manufacturing environments demand inspection systems that can operate reliably despite challenging conditions like vibration, dust, temperature fluctuations, and variable lighting. Industrial cameras designed specifically for quality control applications feature robust housing, specialized optics, and sophisticated image processing capabilities that allow them to deliver consistent results even in these difficult environments.
The shift toward smart cameras—those with built-in processing capabilities—has also simplified implementation for many manufacturers. These integrated devices can perform image analysis directly, reducing the need for separate computing hardware and simplifying communication with production control systems. Some advanced models can even adapt their inspection parameters automatically based on changing production conditions.
According to recent industry analyses, the industrial camera market for quality control applications is growing at approximately 7.8% annually, with particularly strong adoption in electronics, automotive, and pharmaceutical manufacturing. This growth reflects both the increasing importance of visual quality inspection and the improving cost-effectiveness of camera-based solutions compared to traditional methods.
Line scan vs. area scan cameras for continuous production
When designing a vision-based quality control system, one of the most fundamental decisions is choosing between line scan and area scan camera technologies. Each approach offers distinct advantages depending on the specific inspection requirements and production environment.
Area scan cameras capture complete images in a single exposure, similar to conventional photography. They excel at inspecting discrete objects that can be positioned within the camera's field of view, such as electronic components, packaged products, or mechanical assemblies. With resolutions now regularly exceeding 20 megapixels, these cameras can detect extremely fine details across relatively large areas.
Line scan cameras, by contrast, capture just a single line of pixels at a time, building complete images as products move past them on conveyor systems. This approach is ideal for continuous production of materials like textiles, paper, metal sheets, or any product with a continuous flow. Line scan systems can inspect objects of unlimited length and often achieve higher effective resolutions than area scan alternatives for the same cost.
- Area scan cameras: Best for discrete objects, 3D inspection, and complex pattern recognition
- Line scan cameras: Ideal for continuous materials, cylindrical objects, and very high-speed production
- Hybrid systems: Combining both technologies for comprehensive inspection of complex products
Manufacturing facilities increasingly implement hybrid systems that combine both technologies to address different aspects of quality control within the same production line. For instance, a textile manufacturer might use line scan cameras to inspect fabric for weaving defects during production, followed by area scan cameras to verify the quality of cutting and stitching operations.
High-speed CMOS sensors for Sub-Millisecond capture
The advent of advanced CMOS sensor technology has dramatically increased the speed at which quality inspection can occur. Modern industrial cameras equipped with high-speed CMOS sensors can capture crisp, detailed images with exposure times measured in microseconds, effectively "freezing" objects moving at high speed on production lines.
These ultra-fast capture capabilities allow for inspection of products moving at rates that would have been impossible to assess visually just a few years ago. Electronics manufacturers can inspect thousands of components per minute as they fly past cameras on high-speed pick-and-place machines. Food processors can verify the quality of products passing inspection points at speeds exceeding 100 feet per minute.
The latest generation of high-speed CMOS sensors also offers improved light sensitivity, reducing the need for intense illumination systems that could potentially damage light-sensitive products or create uncomfortable working conditions. Many can now capture clear images under normal production lighting, simplifying integration into existing manufacturing environments.
Perhaps most importantly, these sensors maintain high image quality even at extreme capture speeds. The global shutter
functionality found in premium industrial cameras ensures that all pixels are exposed simultaneously, eliminating the distortion that would otherwise occur when capturing fast-moving objects. This precision is essential for dimensional measurements and defect detection at production speeds.
Thermal imaging integration for non-visible defect detection
Thermal imaging technology has emerged as a powerful complement to visible-light inspection systems, enabling the detection of defects and quality issues that remain invisible to conventional cameras. By measuring the infrared radiation emitted by objects, thermal cameras can reveal temperature variations that often indicate underlying problems in products or processes.
In electronics manufacturing, thermal imaging can identify components that are overheating during functional testing, potentially indicating defective parts or assembly errors. The technology can detect temperature differentials as small as 0.05°C, making it extremely sensitive to subtle variations that might signal quality issues.
For pharmaceutical and food products, thermal imaging helps verify proper sealing of packages, as temperature differences often reveal gaps or inconsistencies in seals that could compromise product integrity or shelf life. These systems can inspect hundreds of packages per minute without physical contact, eliminating the risk of contamination or damage.
Thermal imaging inspection systems have been shown to increase detection rates of certain internal defects by over 200% compared to traditional visual inspection methods, particularly for composite materials and sealed containers.
The integration of thermal imaging with conventional visual inspection creates multi-modal quality control systems that provide much more comprehensive defect detection than either technology alone. Modern quality control platforms increasingly incorporate data fusion techniques that combine insights from different imaging modalities to make more accurate pass/fail decisions.
Polarization cameras for stress and surface analysis
Polarization is a property of light that conventional cameras ignore but that contains valuable information about material surfaces and internal stresses. Specialized polarization cameras capture this information, revealing details about surface characteristics, material composition, and mechanical stress that would otherwise remain hidden.
When light reflects off a surface, its polarization state changes in ways that depend on the material properties and surface conditions. Polarization cameras analyze these changes to detect surface defects like scratches on highly reflective materials, identify material differences that appear identical in conventional images, or even visualize mechanical stress patterns within transparent objects like glass or plastic.
In automotive manufacturing, polarization imaging helps verify the quality of painted surfaces by revealing subtle imperfections that might be invisible under diffuse lighting. The technology can detect variations in paint application, underlying surface defects, and areas of potential delamination before they become visible problems.
For plastic product manufacturers, polarization cameras can visualize internal stresses in molded components—often appearing as distinctive colored patterns when viewed through polarization filters. These stress patterns can identify parts likely to fail prematurely, allowing manufacturers to adjust their molding processes to produce more durable products.
As with other advanced imaging technologies, the interpretation of polarization data requires specialized algorithms. Many systems use machine learning techniques to categorize polarization patterns and identify those associated with quality issues, automating what would otherwise be a complex analytical process requiring expert interpretation.
Ai-powered image processing for quality metrics
The true power of modern quality control imaging solutions lies not just in capturing images but in extracting meaningful information from them. AI-powered image processing has transformed how manufacturers interpret visual data, moving beyond simple defect detection to comprehensive quality analysis that drives process improvement.
These advanced processing systems use convolutional neural networks and other deep learning architectures to analyze images in ways that mimic—and often exceed—human visual cognition. Unlike traditional image processing that relies on explicitly programmed rules, AI systems learn to recognize patterns and anomalies from examples, continuously improving their accuracy as they process more images.
One of the most significant advantages of AI-powered image processing is its ability to handle variation. Traditional rule-based systems often struggle with natural variations in products or lighting conditions, generating false positives that reduce efficiency. AI systems can learn to distinguish between acceptable variations and genuine def
ects. This adaptability is particularly valuable for inspecting natural products like wood or leather, where every item naturally varies in appearance but must still meet quality standards.
The metrics generated by AI-powered image processing go far beyond binary pass/fail determinations. These systems can quantify quality on continuous scales, track trends over time, and correlate visual characteristics with functional performance. For example, an AI system might score the consistency of pharmaceutical tablet coating on a 0-100 scale, allowing manufacturers to identify subtle process drift before it results in rejected products.
Perhaps most importantly, AI-powered quality metrics provide actionable insights for process improvement. By analyzing patterns in detected defects, these systems can identify likely root causes—whether a specific production line, time of day, material batch, or operator is associated with higher defect rates. This information allows manufacturers to address underlying issues rather than simply rejecting defective products.
The implementation of AI-based image processing typically follows a phased approach, beginning with supervised learning on labeled datasets before transitioning to more autonomous operation. As the system processes more images and receives feedback on its decisions, its accuracy continuously improves, often reaching expert-level performance within months of deployment.
Leading manufacturers now integrate these AI quality metrics directly into their production dashboards and decision support systems, creating a closed-loop process where quality insights automatically trigger maintenance activities or process adjustments. This integration is key to achieving the full potential of imaging-based quality control systems.
Cloud-based quality control platforms and visualization tools
The shift toward cloud-based quality control platforms represents one of the most significant advances in how manufacturers manage visual inspection data. These platforms centralize image storage, processing, and analysis while making quality insights accessible to stakeholders across the organization and throughout the supply chain. The result is unprecedented visibility into product quality and production trends.
Cloud platforms offer several distinct advantages over traditional on-premises quality control systems. They provide virtually unlimited scalability for storing the massive image datasets generated by continuous inspection, enable remote monitoring of quality metrics from anywhere in the world, and facilitate collaboration between quality teams across different manufacturing locations. Many also offer subscription-based pricing models that reduce capital expenditure requirements.
Advanced visualization tools transform complex quality data into intuitive dashboards and reports that highlight critical issues. Heat maps can show defect distributions across products, trend charts track quality metrics over time, and comparative visualizations highlight differences between production lines or facilities. These tools make quality insights accessible not just to specialized analysts but to everyone from production supervisors to executive leadership.
Cloud-based quality control platforms have reduced the time to identify and address systemic quality issues by an average of 73%, according to a 2023 survey of manufacturers across industries. This dramatic improvement stems from the ability to analyze patterns across millions of inspection images simultaneously.
Security remains a priority for these platforms, with leading providers implementing robust encryption, access controls, and compliance features to protect sensitive manufacturing data. Many now offer private cloud deployments that combine the benefits of cloud architecture with the security of on-premises infrastructure, addressing concerns in industries with strict data protection requirements.
The integration capabilities of these platforms are equally important, with APIs allowing quality data to flow seamlessly between inspection systems, manufacturing execution systems (MES), enterprise resource planning (ERP) software, and customer quality portals. This connectivity ensures that quality insights drive action throughout the organization and its extended value chain.
Implementation case studies: ROI and defect reduction metrics
The transformative impact of imaging-based quality control is perhaps best illustrated through real-world implementation case studies. Across diverse industries, manufacturers are achieving remarkable returns on investment while dramatically reducing defect rates. These success stories provide valuable benchmarks and implementation insights for organizations considering similar quality control enhancements.
The financial returns from these implementations typically come from multiple sources: reduced quality control staffing costs, lower scrap and rework rates, decreased warranty claims, and the ability to produce more consistently high-quality products that command premium pricing. Many manufacturers report payback periods of 12-18 months for comprehensive imaging systems, with ongoing benefits that continue to accumulate year after year.
Beyond the financial metrics, these case studies highlight important implementation lessons. Successful deployments typically involve cross-functional teams from quality, operations, IT, and engineering. They begin with clearly defined quality standards and inspection criteria, incorporate thorough validation processes, and include comprehensive training for operators and maintenance personnel who interact with the systems.
Perhaps most importantly, these implementations demonstrate that imaging-based quality control is not just about catching defects—it's about fundamentally improving manufacturing processes. By providing detailed, data-driven insights into quality issues, these systems enable manufacturers to identify and eliminate root causes, progressively reducing defect rates over time rather than simply separating good products from bad ones.
Automotive manufacturing: Mercedes-Benz paint inspection system
Mercedes-Benz's implementation of an advanced paint quality control system at their Sindelfingen plant represents one of the most sophisticated applications of imaging technology in automotive manufacturing. The system combines high-resolution cameras, multi-angle lighting, and AI-powered defect detection to inspect the painted surfaces of vehicle bodies with unprecedented precision.
The implementation includes more than 100 cameras positioned strategically around each vehicle, capturing images as cars move through the inspection tunnel. Specialized lighting alternates between different angles and intensities, revealing surface imperfections that might be invisible under constant lighting conditions. The AI analysis engine processes over 100 gigabytes of image data per vehicle in real-time, identifying defects as small as 0.2mm.
Since implementing this system, Mercedes-Benz has reported a 58% reduction in customer-reported paint defects, contributing significantly to their consistently high quality rankings in J.D. Power surveys. The system has also reduced the need for manual inspection, allowing skilled technicians to focus on addressing identified issues rather than searching for them. Perhaps most importantly, the data generated by the system has allowed paint shop managers to identify and eliminate sources of defects, resulting in a 31% overall reduction in rework requirements.
The Mercedes-Benz case demonstrates how imaging quality control can be successfully implemented even for complex, three-dimensional products with highly reflective surfaces—traditionally one of the most challenging inspection scenarios. Their phased implementation approach, beginning with a single production line before expanding to the entire plant, provides a valuable model for other manufacturers embarking on similar quality control transformations.
Pharmaceutical packaging verification with cognex vision systems
In the pharmaceutical industry, packaging errors can have serious consequences for patient safety, regulatory compliance, and brand reputation. A leading global pharmaceutical manufacturer implemented Cognex vision systems across their packaging lines to verify 100% of products against multiple quality criteria simultaneously. The implementation illustrates how imaging technologies can address critical quality requirements in highly regulated industries.
The system verifies numerous packaging elements including barcodes, expiration dates, lot numbers, and product information on both primary and secondary packaging. High-resolution cameras capture detailed images while OCR (Optical Character Recognition) and code reading algorithms verify text and codes against database records. The system also performs a comprehensive inspection of package integrity, checking for proper sealing, correct label placement, and package completeness.
The implementation has achieved a 99.997% verification rate while operating at speeds of up to 400 packages per minute. This represents both higher accuracy and significantly higher throughput than the previous manual inspection process. The manufacturer has reported a 83% reduction in packaging-related product recalls since implementation, representing millions in cost savings and immeasurable brand protection.
An unexpected benefit emerged in the form of improved production data. The vision system provides detailed metrics on packaging quality trends that allow process engineers to identify and address issues before they become critical. For instance, a gradual increase in minor label misalignments might indicate maintenance requirements for a labeling machine, allowing preventive action before more serious defects occur.
PCB inspection automation at intel's production facilities
Intel's implementation of automated PCB (Printed Circuit Board) inspection systems across their global manufacturing facilities demonstrates the impact of imaging technologies on electronics quality control. With component miniaturization pushing the limits of visual inspection, Intel deployed a multi-tiered imaging solution that combines different technologies to achieve comprehensive quality verification.
The system incorporates optical, X-ray, and 3D imaging technologies to inspect different aspects of PCB quality. Optical cameras verify component placement, polarity, and marking. X-ray imaging examines solder joints and internal connections that aren't visible from the surface. 3D imaging using structured light techniques measures the height and coplanarity of components to ensure proper mounting.
AI algorithms integrate data from these different imaging sources to make holistic quality determinations. The system can identify subtle defect patterns that might indicate process issues, such as systematic solder problems related to specific reflow oven settings or component placement errors associated with particular pick-and-place machines.
Since full implementation, Intel has reported a 72% reduction in field failures related to PCB defects while increasing inspection throughput by over 300% compared to previous methods. The economic impact extends beyond the immediate quality improvements—by identifying subtle defects earlier in the production process, the system has reduced rework costs by approximately 47% and virtually eliminated complex repairs that would previously have occurred at later assembly stages.
Intel's approach illustrates how different imaging technologies can be combined into a unified quality control solution that addresses multiple inspection challenges simultaneously. Their implementation also highlights the importance of integrating quality data with process control systems to ensure that insights from inspection directly inform manufacturing parameters.
Food safety compliance using TOMRA sorting solutions
Food safety represents one of the most critical applications of imaging-based quality control, with contamination or defects potentially impacting consumer health. TOMRA Sorting Solutions implemented a comprehensive food inspection system for a major processed food manufacturer, combining multiple imaging technologies to ensure both safety and quality standards.
The system incorporates visible-light cameras, NIR (Near-Infrared) spectroscopy, and X-ray imaging to detect a wide range of potential issues. Visible cameras identify visual defects such as discoloration or improper sizing. NIR spectroscopy detects chemical composition anomalies that might indicate spoilage or foreign material. X-ray imaging identifies dense contaminants like glass, metal, or stone that might be invisible to other detection methods.
Machine learning algorithms fuse data from these different imaging modalities to make real-time accept/reject decisions for individual food items moving on high-speed conveyors at rates exceeding 15 tons per hour. The system can distinguish between critical safety issues that require product rejection and minor quality defects that might be directed to different grading channels or processing streams.
The implementation has resulted in a 99.9% detection rate for foreign materials while reducing false rejections by 72% compared to previous inspection methods. This improvement translates to approximately $2.1 million in annual savings from reduced product waste alone, with additional benefits from enhanced food safety compliance and brand protection.
The TOMRA case study demonstrates how imaging-based quality control can simultaneously address multiple priorities in food processing: ensuring consumer safety, meeting regulatory requirements, reducing waste, and maintaining consistent product quality. It also illustrates how different sensing technologies can be integrated into a unified inspection approach that achieves better results than any single method could provide.